What Is Big Data Analytics?
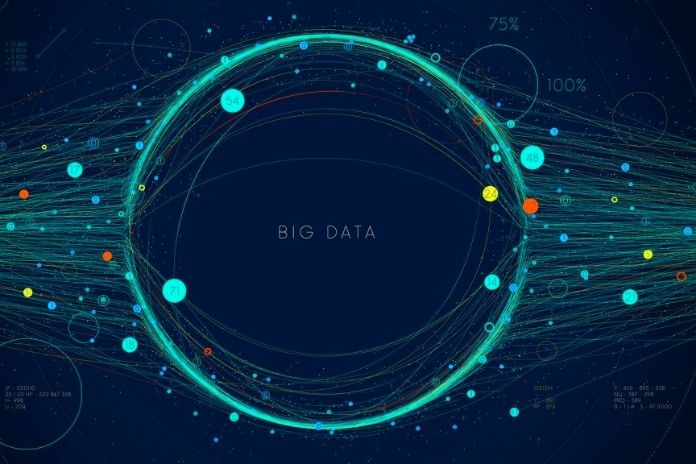
Big data analytics is one of the great revolutions in digital transformation. By examining vast amounts of data for patterns, you get essential consumer insights or optimize production processes, for example. Find out how big data analytics works, what other application areas are, and which technologies are used.
Big Data Analytics
Big data analytics is the generic term for evaluating large, dynamic, diverse amounts of data (big data). This activity comprises three core tasks that build on each other:
- data acquisition
- data preparation
- data evaluation
These three tasks of big data analytics are explained in more detail below.
Data Acquisition:
Due to the large number of different data sources from which big data analytics draws. The main problems are:
- Evaluation of the data source: based on the four essential criteria of information quality: topicality, completeness, correctness, and relevance. Based on the findings of this preliminary analysis, a decision must be made as to whether, how, for what purpose, and to what extent a data source should be used.
- Development of the data source: i.e., retrieval and storage of the data. It is essential to set up an efficient and safe input pipeline. This is usually in a cloud and uses unique storage models such as data lakes.
- Data format for export: or storage. Data formats that data scientists often work with, such as CSV, JSON, or XML, are ideal.
- Update cycles: Some information is no longer up-to-date after just a few hours or days. The data retrieval must be clocked correspondingly high. This, in turn, has consequences for the construction of the input pipeline.
Data Preparation:
Very few data are suitable for analysis in their raw state. They often have to be cleaned up for the study to produce meaningful and correct results:
Missing data must be identified and encoded. Most analysis tools require this step. Outliers, i.e., extreme values far from the mean, should be filtered out because they would distort the analysis results. Faults in the data source or other problems can cause errors in the data set. These cause inconsistencies in the data set or lead to implausible results. These errors are to be tracked down and eliminated in the data processing.
Finally, filtering should narrow the data down to areas relevant to the analysis. This step is essential so that the evaluation software can work efficiently.
Data Evaluation:
The collected and processed data are now evaluated with analysis software. The most critical analysis are:
- Data-Mining: Using methods of analytical statistics such as regression or cluster analysis, attempts are made to identify hidden connections in the database.
- Predictive Analytics: Special statistical analysis models can derive quantitative predictions within a limited framework.
- Machine-Learning: Using large data sets, the software independently recognizes and categorizes specific patterns. Typical examples are movement patterns, images, videos, or texts.
- Text-Mining: Analysis of texts for various purposes: evaluation of customer reviews, automatic text summaries, word analysis, etc.
- Business-Intelligence: Statistical evaluations of key business figures, often presented in dashboards.
You Benefit From These Advantages
The use of big data analytics only makes sense if it contributes to its success. What are the specific advantages? Here are the most common answers to this question:
Master Complexity:
Conventional business intelligence approaches are intended for simple data sets. The evaluation is carried out using descriptive statistics and the analysis of predominantly linear relationships. The aim is to derive business key figures. However, the digitized world is often too complicated for this type of analysis. Considerable data analytics masters complexity better because it also opens up non-linear optimization potential.
Automation Of Business Processes:
Machine learning can make business processes more intelligent and more automated. That saves costs.
Accelerated Product Development:
Companies can optimize and bring innovations to market in much shorter cycles thanks to detailed data about the products and their use.
Optimization Of Purchasing And Warehousing:
If companies consistently record their flows of goods digitally and evaluate them using big data analytics, they can control the required storage and transport capacities more precisely and save costs.
More Safety Thanks To Early Warning Systems:
Big data analytics is suitable for constructing early warning systems based on artificial intelligence, quickly identifying impending disruptions and failures.
Stronger Customer Loyalty:
If Big Data Analytics is used for personalized addressing and individualized services, the company can increase customer loyalty and increase customer value.
Faster Information:
The speed of modern extensive data analytics systems enables companies to analyze their business data in-depth and in near real-time. This allows better decisions to be made faster.
Also Read: How To Develop A Big Data Strategy?